Domain: Environmental monitoring, Renewable energy sources, weather & climate
Lead Participants: Poznan Supercomputing and Networking Center (PSNC), IBT Solutions s.r.l. (IBTS)
Environmental monitoring has been gaining more and more recognition during the last decades. Let us consider flood events, for instance, which are clear manifestations of hydro-geological instability and those with the highest frequency and most devastating and expensive effects. According to the models developed by the University of Amsterdam and the researchers at the UE Joint Research Center (“Nature Climate Change”, 2014), the number of flood events in 2050 could double as a consequence of climate changes. Such an increase, in turn, would have devastating effects on our ability to properly intervene, with a consequent increase in economic impact of more than 500% compared to the current expenses encountered in addressing floods.
Environmental monitoring is often extended with numerical weather prediction (NWP) models, which can be used to predict the occurrence and the range of the floods. These models are not just employed to predict catastrophic events, but also to optimize the behavior of power plants exploiting renewable energy sources (RES) such as wind turbines. Detailed weather predictions are indeed an important source of information for plant operators (e.g. wind speed and direction for wind farms or insolation for photovoltaic systems), as they allow to estimate energy production in selected future time periods. Such knowledge is necessary for owners of RES plants but also for electricity distribution system operators (DSOs) to stabilize the grid and optimize incomes from trading energy in the energy market. A 2017 report released by DOE’s National Renewable Energy Laboratory “Enabling the SMART Wind Power Plant of the Future Through Science-Based Innovation”[1] asserts that scientific advances driven by the use of High Performance Computing (HPC) will significantly cut the cost of wind energy. Accordingly, USA is targeting the 20% of its energy needs to be supplied by wind energy by 2030, to reach 50% by 2050. In Europe, wind energy is now the second largest form of power generation capacity. In 2016 wind power covered the 10.4% of EU’s electricity demand. It is expected that by 2030 30% of EU power demand will be provided by wind energy. In both cases, the value of the data is strongly submitted to the timely of the information and the overall reliability of the application processing.
The goal of this use case is two-fold:
- Demonstrate the applicability of weather forecast in two application domain, i.e. water level prediction and RES;
- Show that run-time resource management is of paramount importance to achieve reliability and to satisfy timing-related performance in a cost-effective manner.
First application domain: H2Observer
IBT Solutions s.r.l. (IBTS) is designing a set of in-field deployable platforms and a dashboard application (see figure below) to keep the status of water basins under control, targeting regions with a large population (in the scale of millions of people). The long-term goal is not only to monitor the status of rivers and canals, but also to acquire and manage a complete set of information in order to:
- Improve the reaction speed to critical conditions such as floods by exploiting weather forecasts;
- Make the application running on the server more reliable;
- Create a repository with historical data for analysis and forecast collected through a flexible, easily deployable and low-cost sensor station.
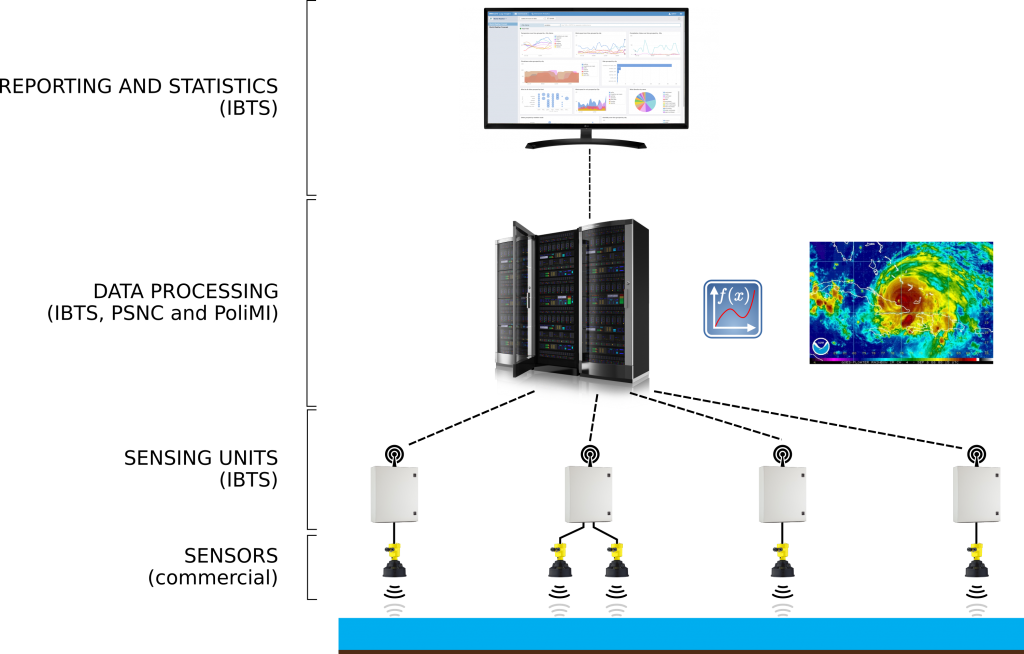
- Reach the customers by moving beyond the system integrator role, i.e. by providing a secure and flexible solution in a bundle, covering the sensing unit and a novel reliable application layer;
- Create a control dashboard for different end users (managers, technicians, geologists, other customers, public bodies …), capable to keep under control not only the parameter to be monitored but the overall status of the apparatus with respect to the expected rainfalls and weather conditions;
- Exploit the RTRM to ensure timing QoS and application reliability.
Interestingly, weather predictions are not merely used to enhance water level predictions, hence flooding. The data coming from the on-field sensors (water level, humidity, speed and direction of wind, etc.) can also be used to enhance the precision of weather predictions themselves, thus creating a very useful synergy.
Second application domain: EULAG
Author: Michal Kulczewski
Even though the wind industry and the wind energy technology have advanced dramatically in recent years, there is still a lot of uncertainty in the science behind wind plant physics. This uncertainty limits the future developments of wind turbines and power plants. In order to understand, control and predict the performance of wind plant, it is crucial to understand physical phenomena such as regional weather systems and the wind flow that passes over wind turbines. Recent advances in HPC allowing more sophisticated physical phenomena to be modeled, make it possible for us to study the wind in new ways. So far, most wind energy DSOs rely on a statistical approach and a general weather forecast to predict the amount of energy produced by their wind turbines. The statistical approach is based on an often manual analysis of the amount of energy produced, which tries to find a correlation between the forecasted weather conditions and the real measurements.
PSNC applies High Performance Computing (HPC) simulations to predict the energy produced by renewable energy sources (RES). It requires reliable, time-constrained, but also cost-effective execution. In the workflow developed by PSNC, the idea is to combine global models’ forecasts with local weather simulations using the EULAG model. Data obtained from mesoscale forecasts is put into separate numerical model suitable for predictions at local scale. Initial data with resolution of several kilometers is supplemented with topography data, which are much denser (horizontal resolution of the order of meters). It allows to simulate local weather with denser computational mesh, also in vertical direction. In addition to this, the simulation produces results with smaller time intervals compared to the mesoscale models. The proposed use case can therefore serve in a twofold way. For the existing wind turbines, the simulation provides a more detailed weather forecast to maximize the outcome of the power plant. For not-yet existing plants, this scenario may help in finding the most optimal localization of the wind turbines to maximize benefit from local topography and weather conditions. The first approach has been already used in cooperation with large Polish DSO.
It is estimated that more than one billion people are exposed to outdoor air pollution annually, which is linked to up to one million premature deaths and one million pre-native deaths each year. Increased air pollution in major cities is caused by rapid urbanization, especially in developing countries. Air pollution in urban areas comes from manufacturing market, in-house thermal appliances, power plants, though 90% is attributed to vehicle emissions brought by high number of older vehicles and poor vehicle maintenance. In order to improve the quality of life in such polluted urban environments, it is important to monitor and predict the air quality.
PSNC simulations software uses HPC simulations to predict and forecast air quality in urban areas, modelling NOx, SOx, PM2.5 and PM10 concentrations in particular. The EULAG model allows different scales to be modeled, taking into account:
- Weather conditions, including forecasts;
- Different emission types: point, line, area, including vehicle types, emission based on land cover;
- Complex urban topography;
- Season differentiation.
The hiqh-quality, advanced visualization is applied to present the simulation results (figure above).
The main goal of PSNC in the project is to ensure reliable and in-time (but also efficient) air quality modeling and execution of energy production prediction in order to allow fine-grained optimization in future smart grids. This could be combined with power grid simulations available in the consortium. In order to achieve this, numerical weather prediction model has to run in an environment that is capable of providing results within given time constraints with respect to the reliability: possible hardware failures, quality of the results, different accelerators being used, different priorities of the jobs running in the same environment etc.
[1] Dykes, Katherine et al., “Enabling the SMART Wind Power Plant of the Future Through Science-Based Innovation”, National Renewable Energy Laboratory (NREL), Golden, CO (United States), 2017.